Mathfi-Legal
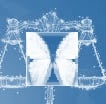
Log in/Register Request a Demo Instruction Manual
A next generation AI-powered service helping legal experts to make better informed decisions whenever a binary or multi-class prediction or classification is required. For example:
1. Crime prediction and prevention: Butterfly AI can assist law enforcement units in predicting crime occurrences at any given location and time associated with a probability/certainty/risk metric in order to take pre-emptive and preventative actions to protect the society and reduce the crime rates. Having recorded data features of tens of thousands of past crimes in a region including post code, x and y geo-coordinates (i.e., the exact location of the past crimes), the district (e.g. downtown, suburb, etc.), the presence distribution of people with criminal records in that neighbourhood when the crime happened, date, day of the week, exact time of the crime, if a person with criminal record is present at that time within the 500m of the given coordinate and having identified the most similar criminals to that criminal (similar in terms of criminal record, crime category, age, gender, city of residence, income, job status drug addiction, nationality, etc.) that have been present in a similar time in that location and having established the number of crimes those similar criminals have committed in past in each crime category within 500m radius of the given geo-coordinates (similar criminals can be identified through a knowledge graph as an example), resolution (arrested, charged, no arrest, convicted, no conviction, open, etc.), special events during that time (e.g., festivals, concerts, etc.), weather, temperature, closest commercial establishments to the location of the crime (cinema, nightclub, football stadium, food market, major bank, beach, etc.), transport or non-transport crime, reported by public or not, level of health damage, amount of financial loss, number of people present in 200m radius from the crime location, number of visible public security cameras in that location, the visibility level of the cameras, light intensity on the crime spot when it happened, frequency of the law enforcement commute in that area, the presence of the law enforcement units in the vicinity of that area when the crime happened, similar crime rates in that neighbourhood, average credit rating of people present in that area during that time, number of the escape routes that criminals can take (underground, public park, etc.) from the crime spot, the macroeconomics data features of that district in terms of average income per household, addiction levels, how affluent the area is, number of people on benefit, unemployment level, inflation, debt level, and other potentially relevant data features and having verified and labelled all those past crimes into correct category (e.g., theft, GBH, assault, etc., the label and target of the prediction), for a new given location and during next couple of hours, predict what sort of crime is likely to happen and with what probability/certainty/risk metric provided that all those data features, described above, are available during the prediction process.
2. Detecting accounting/tax fraud: Butterfly AI can help auditors and relevant authorities to detect accounting or tax frauds by detecting anomaly patterns within data. Knowing the key data features of hundreds of past audited accounts including total current assets, trade account payables, total assets, total common/ordinary equity, cash and short-term investments, cost of goods sold, common shares outstanding, total debt in current liabilities, long-term debt issuance, total long-term debt, depreciation and amortization, income before extraordinary items, total inventories, other investment and advances, total short-term investments, total current liabilities, total liabilities, net income (loss), total property, total plant and equipment, total preferred/preference stock (capital), retained earnings, total receivables, sales/turnover (net), sale of common and preferred stock, income taxes payable, total income taxes, total interest and related expense, fiscal annual price close, WC accruals, RSST accruals, change in receivables, change in inventory, percentage of soft assets, depreciation index, change in cash sales, change in cash margin, change in return on assets, change in free cash flows, retained earnings over total assets, earnings before interest and taxes over total assets, actual issuance and book-to-market and any other potentially relevant data features and having verified, confirmed and labelled each one of those past audited account cases as (0: Not Fraudulent and 1: Fraudulent) i.e. labels and targets of classification and detection process, decide whether a new audited account is fraudulent or not (and also present the probability and certainty of fraud) provided that the data features (described above) are available to the classification and detection process.
3. Predict the litigation threat: Knowing the detailed data features of the past litigation cases including the coordinates of litigation in terms of location, the financial loss value involved, the damage level (for example high, low, medium), liabilities, insurance value, policy value, the case category, time, and many other useful and relevant data features and having the outcome of those past similar cases as of went to litigation or not, predict whether there is a litigation threat for current case. The certainty factor (as of probability) generated by Butterfly AI can help lawyers make better informed decisions by assessing and sanity checking their own judgment and by considering the prediction certainty generated by Butterfly AI as a risk level of litigation.
Or any other binary or multi-class prediction or classification use cases required by legal experts to make decisions.